The AI Summit: Real world AI
A lot changes in a year. Last year’s AI Summit felt largely hypothetical in parts and focused on the potential of the technologies. This year, the conversations are far more focused on use cases, pathways, and challenges to implementation and, as typically follows, the evolving regulatory frameworks.
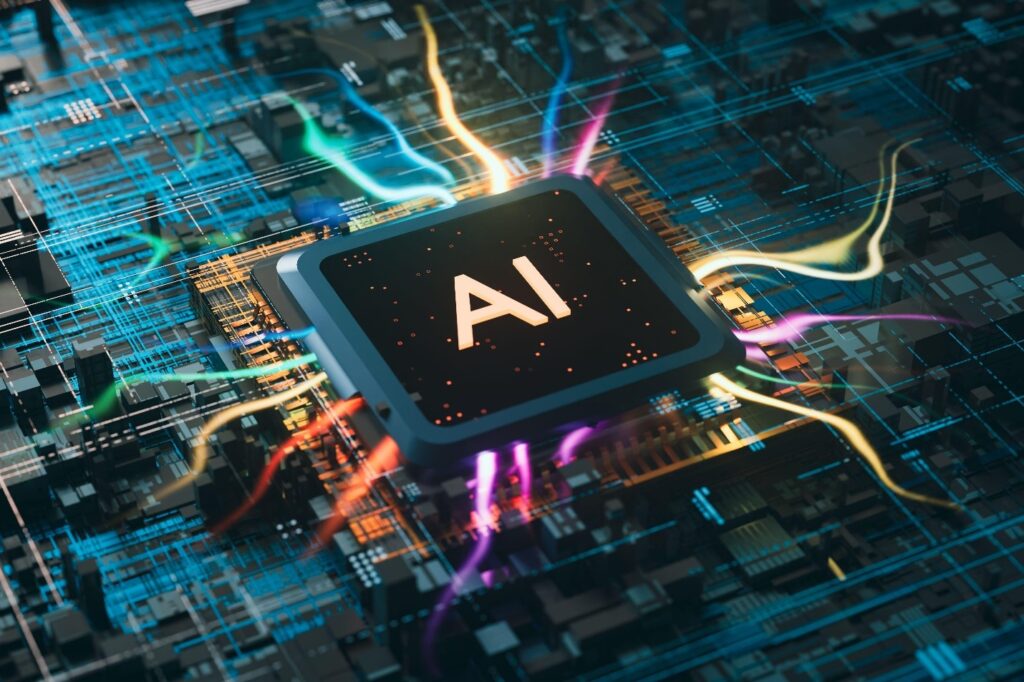
The AI Summit: use cases, pathways, challenges to implementation, and the evolving regulatory frameworks
Colin Jarvis, distinguished architect at OpenAI, kicked off the summit with a with a look at the evolution of language models. To summarise, things are moving fast, very fast. With that in mind, his advice is that companies need to build for what is coming next and not what is currently available.
And what is coming next? Multimodal models, which incorporate different types of data (text, images, audio and video) will change the way we interact with AI and will be far more flexible in terms of the output. They will also be more responsive to and, understanding of, particular contexts.
What is also coming next are considerations around governance and regulation. If that isn’t built in from the start, challenges will follow. The EU AI Act, for example, stipulates fines of up €35 million or 7% of a company’s annual turnover for non-compliance of high-risk AI systems, as flagged by Ritika Gunnar, general manager, data and AI at IBM.
What was particularly interesting were observations from both Jarvis and Gunnar about the future direction of travel and how GenAI tools can and should be constructed to deliver results for businesses. That potential development sits within enterprises.
If, let’s say, GenAI models have accessed 100% of publicly available data and everyone is using the same models, built on the same data, what will separate a company from the herd? What is the differentiator?
The answer to that question is enterprise data. Current (public) models only incorporate less than 1% of enterprise data and it only by leveraging private (enterprise) data that compelling and valuable propositions can be built. As Jarvis noted, you don’t differentiate yourself by accessing the same information that everyone else is able to access. What will set tools and use cases apart are the user experience built on the back of the data included and, ultimately, the products and services offered.
As Harry Nilsson sang, You put the lime in the coconut and drank ‘em bot’ up.
In this case, if the coconut is your base model, as Gunnar demonstrated, you need to be confident about its performance and transparency, the broad commercial rights and indemnification. If things go wrong, the impact on reputation is immediate and the cost of falling foul of regulators in Europe, as noted previously, is potentially substantial.
However, the power of an enterprise’s lime, ie. its own data, is what will deliver the products and services of the future. Whatever model comes out of that mixture will have to be governed and it is the lack of governance models that is the number one inhibitor to implementing GenAI solutions within a given organisation.
When it comes to the use of GenAI in financial services and other highly regulated industries, as Paul Dongha PhD, group head of data and AI ethics at Lloyds Banking Group demonstrated, caution and care need to be front of mind.
He also highlighted two issues that have perhaps not been discussed enough – the cost of a large language model (LLM) query and the sheer magnitude of energy and compute cost to run LLMs. A Google search costs roughly 1 cent, generating 1.6 cents in revenue, whilst a ChatGPT query can set you back almost 10 cents. And when it comes to energy, the fact that Amazon Web Services (AWS) recently purchased a nuclear power plant to power one of its data centres gives you a clue as to the enormous amount of energy required.
As the use of AI technologies grow and GenAI tools are rolled out and continue to evolve from the hypothetical to the realisable, all of the real-world considerations of other technologies (ie. the internet) are being asked of AI. Is it fair? Is it safe? Is it transparent? Is it a net gain for economies and societies around the world? Those are questions that still need answers.