Unpacking the hype: Generative AI in banking and finance
The year 2023 will go down as the year generative AI became fashionable. From the overwhelming response to OpenAI’s ChatGPT to an increasing adoption of the technology among businesses across the world, generative AI has truly stepped into the spotlight.
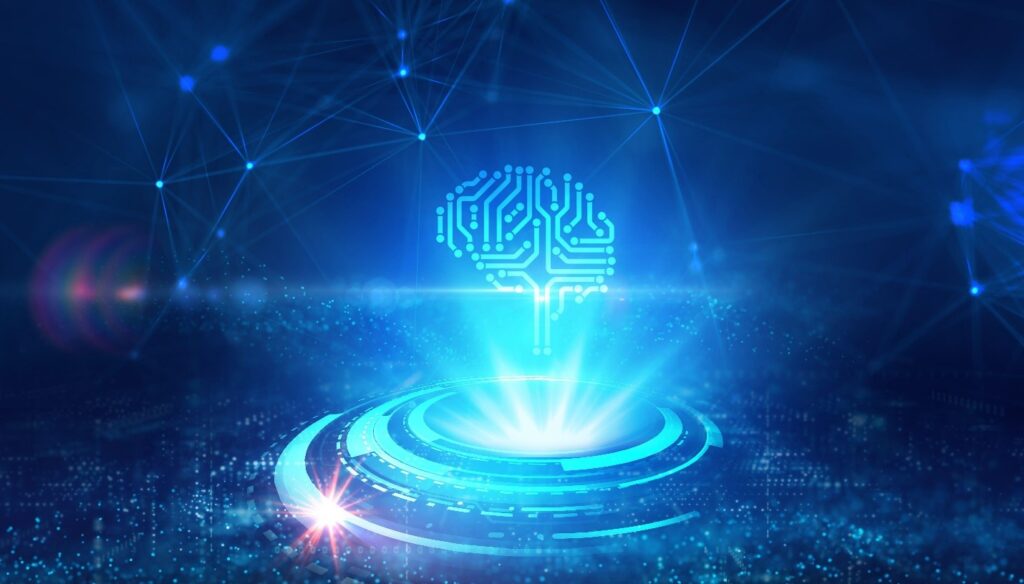
Generative AI has boomed in 2023, with businesses across all sectors looking to leverage the technology
If the surge of funding into AI and the long queue of AI patents waiting to be filed are anything to go by, then the hype is far from over. And while clouds of concern surround this topic, just as they do with anything that is new and unknown, the one thing certain about generative AI is the bullish sentiment around it.
“Unlike other technologies that have seen hype cycles, generative AI exhibits clear use cases, has led to the creation of robust solutions, and is developing swiftly. New opportunities will continue to appear. This technology is poised to be transformative across nearly every sector,” the latest Mastercard Signals report reads.
And it’s not just Mastercard that expects the coming years to see a “widespread integration” of generative AI across all possible sectors.
Decoding the hype
The world of finance is no stranger to the capabilities of artificial intelligence, with many financial institutions engaging with the technology years before it became the centre of attention it is today.
Mastercard attributes the growth of this transformational technology to three factors: availability of data, advancements in computational power, and innovation in deep learning algorithms.
According to a McKinsey report, while only 20% of companies were using AI for at least one business application back in 2017, this number has now grown to 50%.
In EY’s CEO Outlook Pulse Survey for July 2023, 71% of CEOs surveyed said AI is embedded in their transaction strategy processes, with just 5% having no plans to incorporate the tech. Additionally, over half of Europe’s financial services CEOs told EY they have already integrated AI into their capital allocation, with 43% planning to do so within the next year.
A new study from FIS, which surveyed 800 executives at US and UK financial services companies, also found that UK-based firms were pumping money into AI at higher rates than the US.
“Rarely do we come across technologies that can solve a complex problem so quickly and at scale,” remarks Rohit Chauhan, EVP, AI at Mastercard.
“It has a lot of potential, but the burden is on us to make sure that we leverage it to the tune of its maximum capacity, rather than just use it incrementally to improve the way we have done things in the past.”
Generative AI in banking and financial services
When coupled with informed data consent, Mastercard says that AI in financial services has the potential to “declutter and streamline” processes within areas such as taxation, property registration, and many more.
With a snapshot of an individual’s financial standing, generative AI could very well act as a personal wealth manager, while also integrating bank accounts, investment portfolios, and small business accounts. It could even interact with governmental revenue offices through open banking data methods.
Chauhan highlights some inevitable use cases for generative AI. He categorises them into three buckets – generative AI can help increase efficiency, improve the value proposition of existing offerings, and help us do things that we could never do in the past.
Examples of efficiency include digital assistants or chatbots which have the ability to understand the context of the situation and respond accordingly rather than presenting customers with a “cookie cutter” answer like they did in the past.
“Like asking deeper and deeper questions in the same thread,” Chauhan says.
In terms of enhancing value proposition, Chauhan suggests a future “where interactions with a banking application are not menu-driven but rather a conversation”.
He explains that AI could take on the role of a personal banker (traditionally not available to everyone) in the virtual form that people can converse with and one that can synthesise information and provide customers with accurate responses. Other use cases include training employees and personalisation of the customer experience in banking and financial services, which Chauhan describes as a “huge opportunity”.
Another use case is synthetic data generation, according to Chauhan, particularly when building fraud models.
“From where I sit, fraud can be of different kinds,” he says. “So, when we’re trying to analyse certain types of fraud, the data is extremely thin – maybe because it is not getting caught, or the accuracy of the fraud is not very high.”
In order to train a robust model, institutions need a large dataset, and this is where generative AI comes into the picture to create additional transactions “that look exactly like the fraudulent transactions” which can then be used to build more robust models. This can also be used to help prepare banks on new sets of fraud they have “never seen in the past”.
Examples of adoption are plenty. Just last month, Bud Financial, an open banking and data intelligence firm based in the UK, launched a generative AI core platform that enables banks and financial organisations to transform transaction data into customer insights and enhance personalisation of the customer experience.
Similarly, ForwardLane, which provides AI-led intelligence solutions, launched a new generative decision intelligence platform, Emerge, for a similar use case – to help enterprises in the wealth, asset management, and insurance industries find, create, preview, publish, and interact with new or unknown insights while maintaining privacy.
Adoption, despite concerns
The EY report suggests that even though two-thirds, or 63%, of the European financial services CEOs it surveyed remain wary of the unintended consequences of using this technology, they are still embracing the opportunities it has created.
“The biggest risk is not using this technology,” Chauhan states.
This sentiment is echoed by Zor Gorelov, CEO and co-founder of Kasisto, a New York-based company operating a conversational AI platform since 2013.
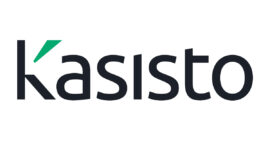
Kasisto has launched Kai-GPT, an LLM specifically designed for banking
“There are some risks that come with generative AI, but banks cannot afford not to work with these (large language) models,” Gorelov says.
“And that’s the sentiment we’re hearing from every bank that we’re engaged with – the risk of not deploying, not experimenting, not trying a technology so transformative, perhaps even as transformative as the internet.”
In a move to adopt generative AI into its platform, Kasisto claims to have launched the “world’s first and only” large language model – Kai-GPT – designed to work in a banking environment. It claims its private model overcomes the issues seen in public LLMs.
“Kai-GPT was built to deliver all the benefits of public LLMs, but also because it’s a private model, it addresses some of the cons that the public model has – namely, protection of personal identifying information, accuracy of responses, customisability, and addressing some of the copyright issues.”
Another worrying risk with the emergence of generative AI is the evolution of fraud.
Chauhan highlights that a downside of this technology is that it “actually put the fraudsters on steroids”. In the wrong hands, AI can be used to create “deep fakes” to trick people and spread misinformation.
“Humans can be quite gullible,” Chauhan says. “Once fraudsters are equipped with this kind of technology, they can pollute the ecosystem and cause new kinds of fraud to pop up which could be so realistic that humans aren’t able to detect them.”
To counter this, Chauhan suggests people should use basic caution when dealing with generative AI applications, and make sure they don’t put any sensitive information into the models.
For financial services, he suggests banks should prioritise customer education to help them become more vigilant when using this technology, as well as set up safeguards to ensure customers are sure they’re talking to a banking agent rather than an impersonator.
What does the future hold?
For Gorelov, who has been working in this space for over a decade, the future lies in “deep transactional experiences”.
“I started this company with the goal to democratise financial services using AI – to help users make sound financial decisions, and for everyone to have the best, most unbiased banker on their shoulder – and I think generative AI creates that opportunity.”
“We envision a world where generative AI systems will become consumer-facing and we’re on the journey but right now, primary interest for banks is focused on deploying private LLM models like Kai-GPT in employee-facing scenarios with the goal of driving employee productivity and efficiency,” Gorelov says.
Chauhan on his part stresses that in the near future, AI is poised to make customer experiences better and the financial ecosystem more efficient, and Mastercard expects this technology to “evolve considerably” in the coming years.
“Even in its nascent form, this powerful technology promises incredible opportunities when combined with human oversight. Safe usage depends on our collective actions as a global community. By embracing generative AI while addressing its risks and challenges, we can step into the future of commerce and make sure it influences the world for the greater good,” Mastercard concludes.