Spoil the magic: machine learning in fintech
Professor Michael Mainelli joins FinTech Futures’ upcoming virtual roundtable gathering, Dock Digital, on 18-19 May, to discuss the burning issues in banking, finance and tech. Here, he shares his candid views, based on decades of hands-on expertise, about the machine learning (ML) applications in banking and finance.
We invite the digital transformation movers and shakers at banks and financial institutions to join him and other senior decision-makers at Dock Digital (it’s free to attend!). Find out more and register here.
Oh, ho, ho, it’s magic, you know
Every futuristic statement about fintech feels compelled to invoke the incantation of “artificial intelligence (AI), big data and blockchain”. Roald Dahl did say, “those who don’t believe in magic will never find it”, but has the financial services industry lost its unsentimental, hard-headed knack for disbelief?
Outside of cryptocurrencies, blockchain has turned out to be either smooth sales patter for consultants, or what it really is, a boring data structure that provides independent and authoritative timestamping.
Big data has turned out to be an enormous headache that consumes much resource yet delivers only sporadic value.
AI is turning out to be a poisoned goblet that turns financial institutions into unethical oppressors chaining historic data to future prejudices.
AI is a field of research developing machines to perform tasks ordinarily requiring human intelligence. AI fulfils Douglas Adams’ definition of technology: “technology is a word that describes something that doesn’t work yet”. Researcher Rodney Brooks complains: “Every time we figure out a piece of it [AI], it stops being magical; we say, ‘Oh, that’s just a computation.’”
In a sense, all complex applications, such as maps on smartphones or predictive text only a few years ago, begin as magic technology to outsiders and rapidly become expected utilities.
Financial institutions rarely want to deploy stuff that doesn’t work yet. Arthur C Clarke’s aphorism, “any sufficiently advanced technology is indistinguishable from magic”, should guide financiers. It’s one thing to talk about the “golden goose” at the core of your organisation, it’s quite another to believe there really is an off-colour fowl making everyone rich.
Nothing in your business should be magic, it’s just nice if it looks like magic to outsiders. We’re talking about machines here.
Breaking the spell
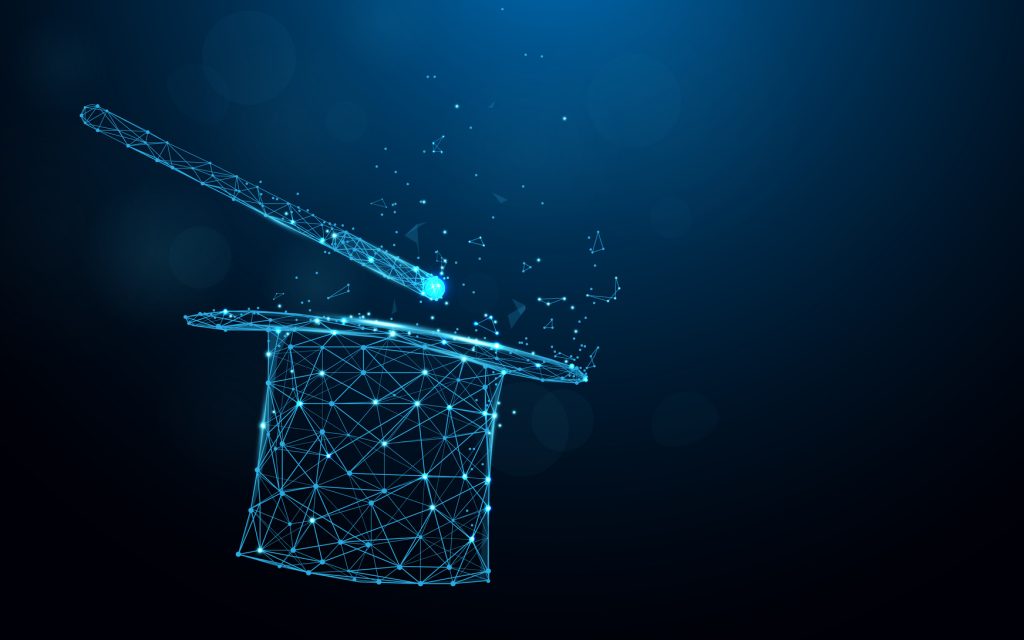
Nothing in your business should be magic, it’s just nice if it looks like magic to outsiders. We’re talking about machines here.
Machine learning (ML) research is related to AI research, developing applications that improve automatically through experience from the use of historic data, but it’s not magic, and not new. Most of the basic techniques of ML were set out by the end of the 1970s, four decades ago. However, the techniques required three things in short supply in the 1970s, namely lots of data, lots of processing power, and ubiquitous connectivity. Data, processing, and connectivity grew enormously since 2000 and ML algorithms have flourished.
Anywhere data, processing and connectivity grow we can anticipate ML to flourish. Take video-conferencing’s recent explosion. Since the COVID-19 pandemic, ML systems can now access huge recorded libraries of human interactions in a controlled environment. They’ve never had this quantity of recorded person-to-person video and audio interaction. Anticipate large-scale simulations of people in video conferences, perhaps an automated secretary and note taker – “Alexa, this comment is not to be minuted” – and suites of ML software to support the real people among the simulations.
Within financial services, in the front, middle, back, and plumbing offices, ML has an important place. It’s one thing to use external ML, e.g. using your smartphone to turn speech into text, quite another to develop the applications yourself. Financial services firms need to develop such applications themselves when they are the source of the data.
Data driving needs statistical guiderails
My firm has deployed ML systems in finance for over a quarter of a century, with some of the original systems still in place. We style our systems as useful for dynamic anomaly and pattern response. ML systems should know what to do with patterns they’ve seen, e.g. routing orders, and inform humans about anomalies, e.g. an unusual trade.
One important thing we’ve learned over that time is that you want to “spoil the magic”. These are machines, not magicians. We’re feeding them training data using old techniques. We then ask questions based on some test data to see how predictive things are. The skill here is not the programming, not the networks; it’s managing the data that fuels ML.
Spoiling the magic means really understanding what’s going on and where it can go wrong. To understand what’s going on means being ruthlessly analytical about describing what the data really is, what the machine is doing with the data, how new data is added, how old data is removed, how everything is calibrated and recalibrated, and when to turn off the machine.
Our experiences in areas such as consumer behaviour prediction or revenue targeting have show that many times people don’t know the sources of their data. In one example, a raft of data on commercial lending had credit ratings in it, but no-one knew where the ratings had come from. It turned out it wasn’t credit rating data at all. Someone, seeing a bunch of AAAs and BBBs assumed it was credit rating data and had relabelled it. Some years later it transpired that the data column was really just a sorting column.
Perhaps the most difficult task is knowing what the machine is doing with the data. A classic tale in ML from University of California Irvine is an application that successfully distinguished wolf pictures from dog pictures, until the researchers gave it pictures of dogs on snow and realised the machine was really picking out canines on snow as wolves; if not then it classified the canines as dogs.
Before deploying one stock exchange application we developed, we kept probing hard on its inability to predict share liquidity. The application was working above the necessary threshold for deployment, but we held back uncomfortably for nearly two months to understand why the remaining mistakes were random. What we uncovered was a hitherto unknown, somewhat shoddy, systematic three-month delay by the exchange in updating industry classifications for its listings.
Adding data, deleting data, and recalibrating ML models are frequently mundane tasks left to the programming team. This can be a mistake. Changes to the training data change the ML application. These tasks are important statistical work. Tasks such as data cleansing or training data selection should be independently checked. There needs to be a clear audit trail on the training data, the test set data, and the states of the algorithm. The team need to have a battery of threshold tests to run every time the training data is refreshed. Too often managers don’t manage “technical tasks”, resulting in cases of organisations floundering, unable to “roll back” to the previous algorithm when the latest version turns out to be failing because of incorrect data within.
Pulling the plug based on your disbeliefs
As ML applications are data driven, they tend to perform poorly when there are rapid changes in the environment. The structure of new data being used for anomaly detection or pattern response doesn’t accord with the environment from the training set.
Predicting heart disease from a set of conditions is something that ML applications can do well. Heart disease conditions do change across the population, but slowly. Financial services are fast-moving and the environment is a complex one of interest rates, exchange rates, indices, and other information interacting dynamically with large degrees of uncertainty in the accuracy of data and the strength of their correlations. Such environmental change rates require commensurate training data refresh rates.
“When to pull the plug” can be a much more important question than “when is the ML application good enough to deploy”. The closer applications are “to the market”, the more likely their off switches need careful attention. We’ve seen trading firms lose more in one day from ML tools than they ever gained over a lifetime of use.
Mason Cooley described one magic trick: “to make people disappear, ask them to fulfill their promises”. By the time we’ve been through the data asking how it fulfils its promises, the magic is thoroughly spoiled. It might be good to close with a Tom Robbins’ observation, “disbelief in magic can force a poor soul into believing in government and business”. Surely that’s what financial institutions need to apply, disbelief.
About the author
Michael Mainelli is executive chairman of Z/Yen Group, the City of London’s leading commercial think-tank.
His book, “The Price of Fish: A New Approach to Wicked Economics and Better Decisions”, written with Ian Harris, won the 2012 Independent Publisher Book Awards Finance, Investment & Economics Gold Prize.