Optimal execution and the human-machine interface
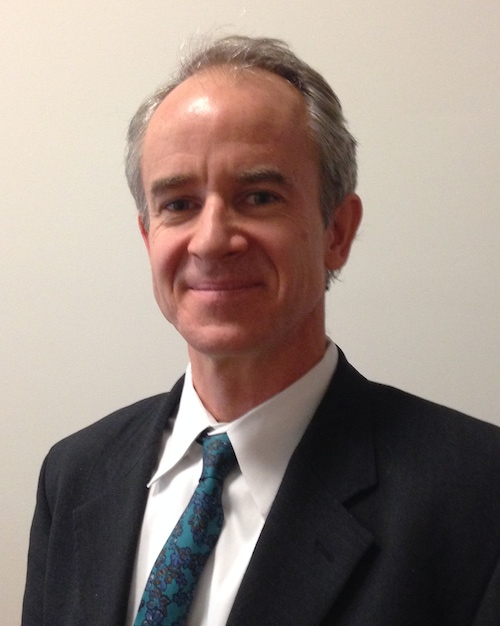
Henri Waelbroeck, Portware: The combination of an intelligent UI with an intelligent trader is the next big step in trading
In recent years we have seen a strong trend for institutional trading desks to leverage their data to improve trade execution results and become more recognised as active participants in portfolio performance. Two important applications of predictive analytics have arisen, both aiming to improve the manner in which trading algorithms are used and controlled. It is important for both the buy-side and their partners to understand how machine learning impacts the business today and how it is likely to evolve in coming years.
The first such application of predictive analytics was algorithm switching. Originally deployed in 2007, the algorithm switching technique is a machine learning solution that leverages the predictability in order flow on a 5-15 minute timescale to dynamically set and adjust algorithm style settings, such as dark pool exposure or aggression, to improve execution efficiency. Buy-side clients select a broker with a high quality algorithm platform and allow the machine to switch between algos in a given broker’s suite or control algo parameters such as tactical limits. Not unlike how cars have benefited from the electronic control of ignition timing and fuel mixture, algorithm switching improves efficiency of algorithmic execution by adjusting style settings as a function of the expected order flow, yielding superior results for both the buy-side trader and her sell-side counterparts.
Of course order flow changes, so a key to making algorithm switching work was the introduction of order flow prediction models: to understand whether trades are more likely to occur on the bid or on the offer side of the spread and whether there is hidden liquidity on either side or in dark pools. A key word here is “prediction”: algorithm settings are going to take effect in the future, not in the past. Technological solutions based on modeling the past, like smart order routers, would not have been effective in setting algorithm instructions on a 5-15 minute timescale.
The second major development in the use of machine learning to optimize institutional trade execution was the introduction of alpha profiling, which aims to answer the following question about every order, both at trade start and on significant events that take place during the execution:
Is a portfolio manager’s order most likely to be aligned with others coming from his peers, creating a challenging competition for access to liquidity and the risk of high opportunity costs if the shares can’t be found before the mispricing is corrected? Or vice-versa, is she acting as a contrarian or “liquidity provider” with this order, in which case there is an opportunity to capitalise on price distortions caused by others?
Answering this question is essential to set the proper execution urgency on an order. Alpha profiling is a machine learning solution intended to predict trade urgency by looking back at historical order creation events from the portfolio manager, enriching the data with the market factors that were known at the time, and determining whether these factors and the order characteristics are consistently predictive of either competitive or contrarian trading conditions over the life of the order. In some cases, the predictive factors reveal behavioral aspects of trading.
In one example, a portfolio manager has a pattern of joining a trade late. After momentum had already developed, the trading desk responds to adverse momentum by executing aggressively but the signal is overplayed and reversion follows. Statistical analysis of such a trade profile reveals the superior performance of a slower execution strategy. While this example may seem simple enough to be recognised by a trader without having to resort to machine learning methods, other cases — or simply the presence of many orders to work at once – require a systematic scan through a library of 20-50 predictive factors to identify consistent urgency profiles that can be expected to generalise in the future.
Taken together, these machine learning tools enhance the trader’s productivity and enable automation of certain classes of orders, releasing the trader to focus on high value trades and other exceptions.
Where do we go from here?
When a buy-side desk has access to ample data and statistical examples, its machines can learn how to use that data to optimally execute some proportion of trades. When a critical mass of data is not available to help understand a given situation, the trader needs to bring his intelligence to bear on the order. To be sure, artificial intelligence has a growing role in institutional trading, but for large institutional desks it is unlikely that trade execution will ever be completely automated: someone needs to be there to answer the phone when unique situations arise, to interface with brokers and other partners, to set strategies, and to oversee the machines.
By their very nature, unique situations are not addressable through statistical methods, but that does not mean machine learning tools are not useful. Traders are exposed to huge amounts of data, displayed on impressive rows of monitors; yet a trader has the same two eyes as the rest of us.
How to process all this information?
The first step is to understand what information is likely to be relevant — information that describes what happened in the past is not necessarily what the trader needs to know to make a trading decision that will play out in the future (but it could be!). An intelligent user interface must identify items of information that are more likely to have a bearing on events that will take place in the window of time when a decision takes effect. Taking this a step further, an intelligent interface can identify intervention opportunities and present them to the trader with the relevant information sources. Here, predictive analytics assists the human trader through more efficient attention management to focus on the most relevant opportunities and not get distracted by the vast amounts of information pouring in that has no actual bearing on optimal decision-making.
The combination of an intelligent user interface with an intelligent trader is very likely the next big step in the continued adoption of advanced data-driven technologies at institutional trading desks. Sell-side institutions who empower the buy side with data, the flexibility to execute trades according to this new generation of analytics, and transparency to support best execution are positioning themselves for success in this new paradigm.
By Henri Waelbroeck, director of research, Portware